Table of Contents
Data science continues to evolve rapidly, driven by technological advancements and the increasing volume, variety, and velocity of data. Emerging trends in data tools are revolutionizing how organizations collect, analyze, and derive insights from data. This article explores key innovations shaping the future of data science tools, their applications, and the impact on industries worldwide.
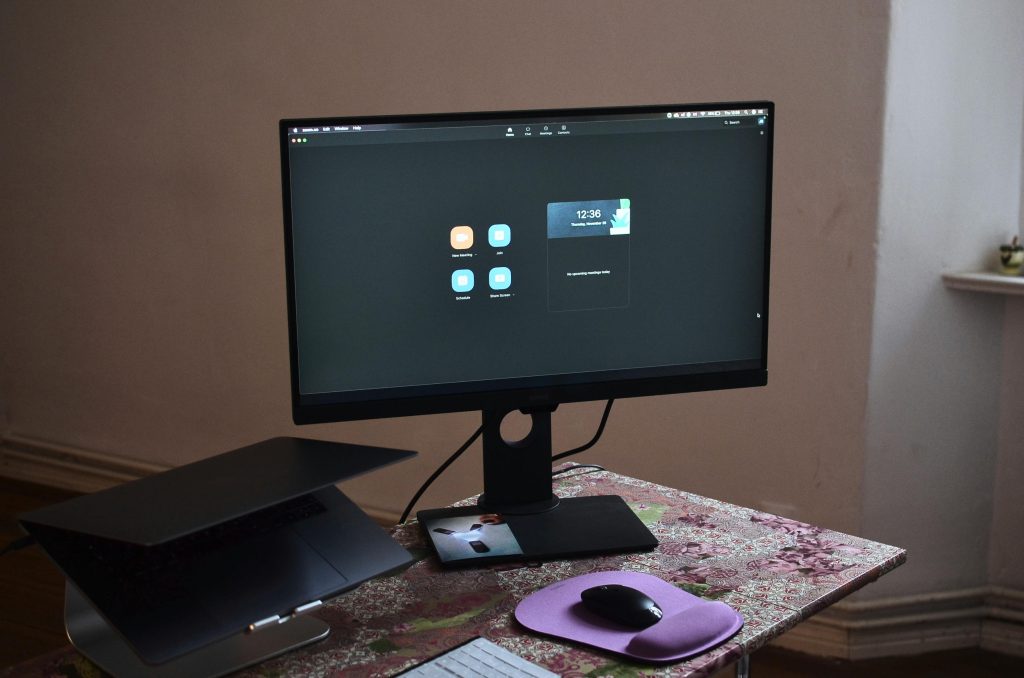
Rise of AI-Driven Analytics
Artificial Intelligence (AI) is revolutionizing data analysis by automating complex tasks and uncovering insights at unprecedented speeds:
1. Automated Machine Learning (AutoML):
- AutoML platforms democratize machine learning by enabling users with minimal expertise to build and deploy models. These tools automate feature engineering, model selection, and hyperparameter tuning, accelerating the model development lifecycle.
2. Natural Language Processing (NLP):
- NLP tools interpret and analyze human language, enabling sentiment analysis, text summarization, and language translation. Advanced NLP models, such as transformers, enhance accuracy and context understanding in data-driven applications.
Cloud-Based Data Platforms
Cloud computing has transformed data storage, processing, and accessibility, offering scalable solutions for businesses of all sizes:
1. Serverless Computing:
- Serverless architectures, like AWS Lambda and Google Cloud Functions, enable automatic scaling and reduced operational overhead. They are ideal for executing event-driven tasks and processing data in real-time without managing infrastructure.
2. Data Lakes and Warehouses:
- Cloud data lakes, such as Amazon S3 and Azure Data Lake Storage, store vast amounts of structured and unstructured data. Data warehouses, like Google BigQuery and Snowflake, provide scalable SQL-based querying and analytics capabilities.
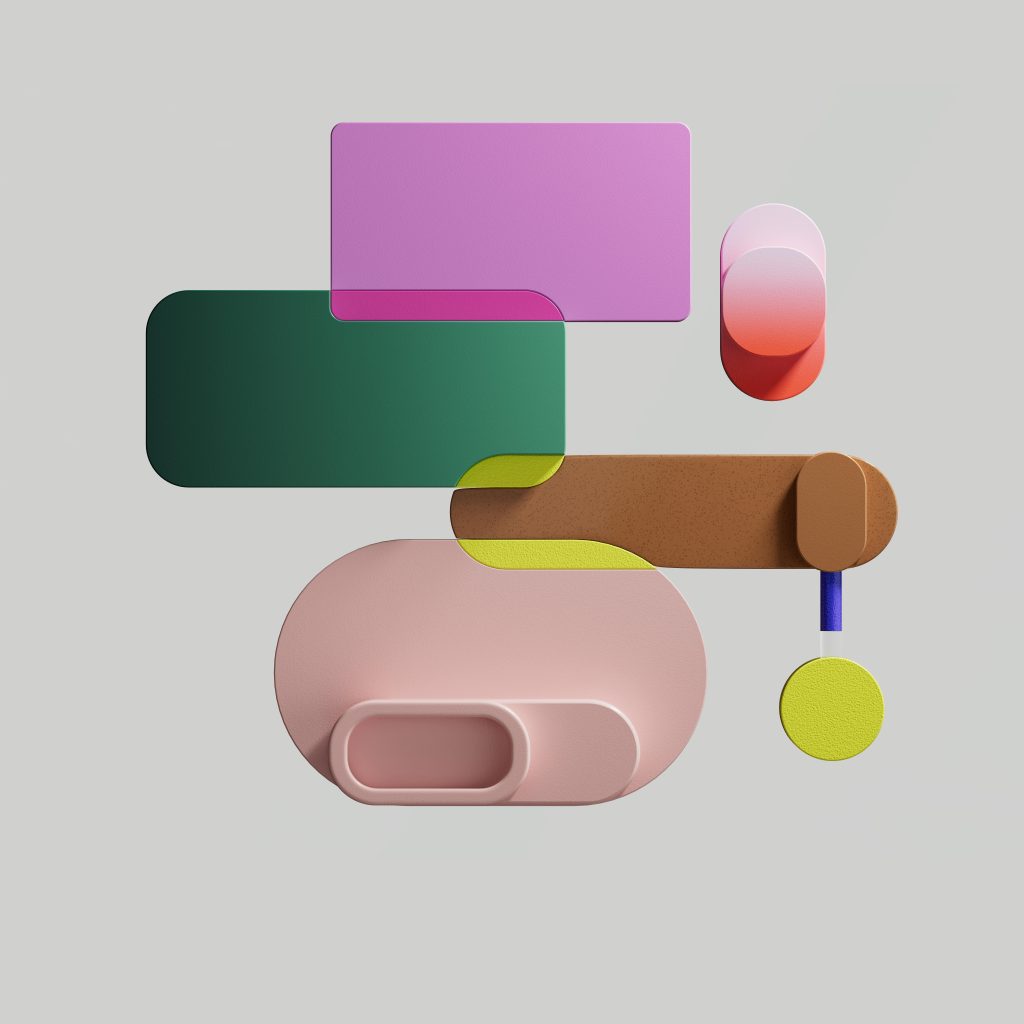
Real-Time Data Processing
In today’s fast-paced digital environment, real-time data processing is essential for timely decision-making and actionable insights:
1. Stream Processing Frameworks:
- Apache Kafka and Apache Flink facilitate real-time stream processing, handling high-throughput data streams and enabling low-latency analytics. These frameworks support continuous data ingestion, processing, and integration with downstream applications.
2. In-Memory Computing:
- In-memory databases, such as Redis and Apache Ignite, store and manipulate data in RAM for faster query response times and real-time analytics. They are crucial for applications requiring rapid data access and processing.
Visualization and Interactive Analytics
Data visualization tools play a critical role in transforming complex data into meaningful insights and actionable visualizations:
1. Interactive Dashboards:
- Tools like Tableau and Power BI enable users to create interactive dashboards and visualizations. These platforms support drill-down capabilities, dynamic data exploration, and real-time updates, empowering users to explore data and uncover trends effortlessly.
2. Augmented Analytics:
- Augmented analytics platforms integrate AI and machine learning algorithms into data analytics workflows. These tools automate data preparation, pattern recognition, and anomaly detection, enhancing data-driven decision-making and reducing time-to-insight.
Security and Privacy Enhancements
As data volumes grow, ensuring data security, privacy, and regulatory compliance remains paramount:
1. Data Governance and Compliance:
- Tools for data governance, such as Collibra and Informatica, manage data quality, lineage, and metadata. They enforce policies, monitor data usage, and ensure compliance with regulatory standards like GDPR and CCPA.
2. Privacy-Preserving Techniques:
- Differential privacy and homomorphic encryption techniques protect sensitive data while allowing meaningful analysis. These methods enable organizations to leverage data for insights while preserving individual privacy and confidentiality.
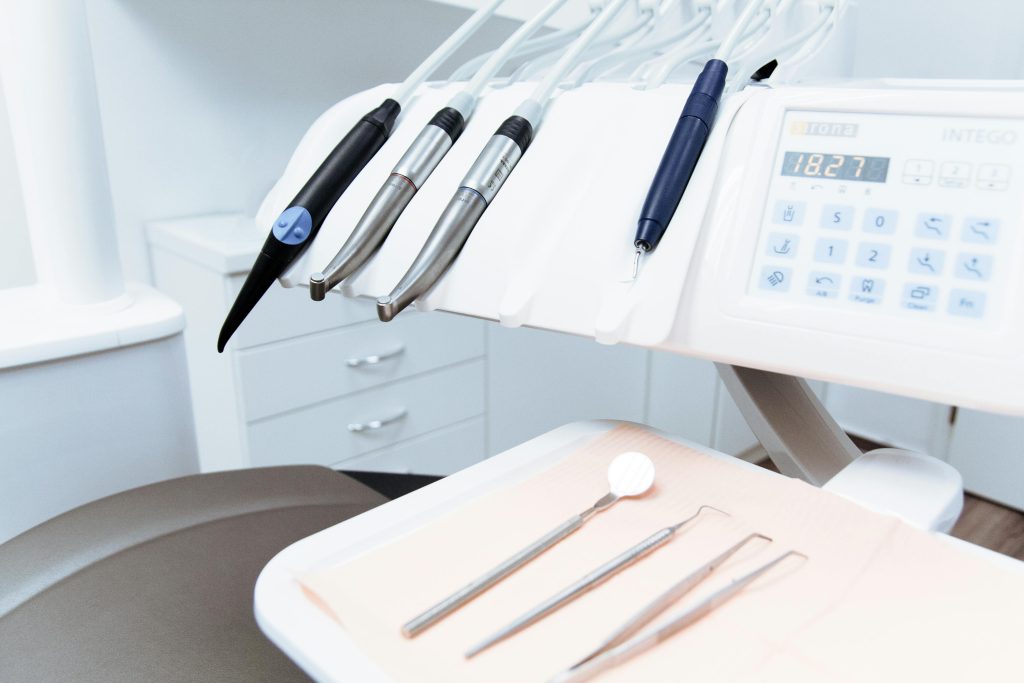
Conclusion
Emerging trends in data tools are reshaping the landscape of data science, empowering organizations to extract actionable insights, drive innovation, and gain competitive advantage. AI-driven analytics, cloud-based data platforms, real-time processing capabilities, interactive visualizations, and enhanced security measures are revolutionizing how data is managed and utilized across industries. As businesses embrace these innovations, they must adapt their data strategies to harness the full potential of data science tools in achieving business objectives and addressing evolving market demands. By staying abreast of these trends and leveraging advanced data tools effectively, organizations can navigate the complexities of modern data ecosystems and pave the way for future growth and success in the digital age.